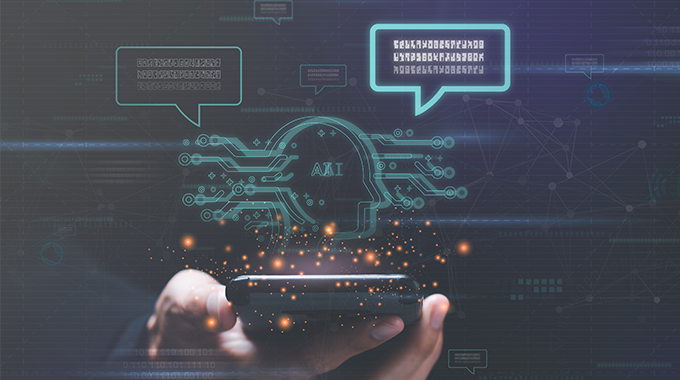
Exploring the Intersection of Machine Learning and Finance
In today’s rapidly evolving financial landscape, the integration of machine learning has become a pivotal point of discussion and exploration. As traditional financial practices intersect with cutting-edge technology, the role of ML in reshaping decision-making processes and predictive analytics has garnered significant attention. Machine learning offers a sophisticated framework for analyzing vast amounts of data, extracting meaningful insights, and making informed decisions in real time. From asset management to risk assessment, ML algorithms are revolutionizing how financial institutions operate and strategize in an increasingly data-driven environment. This transformation not only enhances efficiency but also introduces new possibilities for identifying market trends, managing risks, and optimizing investment strategies. As the financial sector continues to embrace ML capabilities, understanding its implications and potential applications is crucial for staying competitive and adaptive in the modern era of finance. Hence, this Chicago Booth Review article emphasizes the role of machine learning in the finance industry.
According to the article, the integration of machine learning in finance represents a significant evolution, although finance has long been employing machine-learning principles implicitly. The article suggests that researchers like Eugene F. Fama and Kenneth R. French, through their variable selection methods, exemplify how humans are already mimicking what machines are doing today. It also highlights that academic insights, including modern portfolio theory and factor models, have long influenced financial practices. However, the finance industry, particularly some hedge funds, was ahead of academia in adopting machine learning techniques about six years ago. According to the article, academics like Bryan T. Kelly and his colleagues have been instrumental in introducing ML to academic finance, focusing on utilizing advanced ML technologies and alternative data sources.
Despite the growing interest and adoption of machine learning in finance, challenges remain, including interpretability issues due to the black-box nature of ML models and the trade-off between performance and interpretability. The article suggests that finance’s conservative nature also raises questions about the superiority of ML over simpler models in certain contexts, emphasizing the need for caution and theoretical guidance. Nonetheless, the article indicates that ML’s potential in leveraging alternative data sources suggests a promising future in revolutionizing financial decision-making beyond traditional economic approaches.
Machine learning is a growing technology being implemented in almost all domains of the business world. The preceding text highlights a few effective insights about the role of ML in the finance industry.